It’s time for another episode of the DWC book club with the latest recommendations by the water community. Find out what we have been reading and listening to this month.
Katrien Witpas, ARCTIK
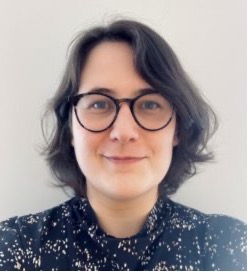
I adore podcasts. Exciting new favourites come and go, but I have an undying love for 99% Invisible. The show examines aspects of the built environment that usually go unnoticed and the thought that went into designing them. It has the peculiar ability of changing how I see the world around me – from wayfinding signs, algorithms and averages, to concrete, lawns, and the different dings and chirps machines and apps make.
There’s rarely a 99pi story that I wouldn’t recommend, but a recent episode on toilets and sewers, ‘Pipe Dreams‘, reminded me of DWC. It zoomed in on several of the issues DWC researchers are tackling; for instance, how sewers can overflow after heavy rain events that are becoming more common in a changing climate.
If you’re a water researcher, the content of this episode will not greatly inspire you, but I think the format and tone might. It’s top-notch science communication that turns research on infrastructure into a compelling story. I’m hoping you’ll come away from the episode with some fun ideas for communicating about urban water research. I know I did.
If you’re not a good listener, I’d recommend the book The 99% Invisible City – A Field Guide to the Hidden World of Everyday Design instead. It only has a short entry about water management, but it’s a fascinating read from cover to cover.
Laura Frølich, DHI group
My role in the DWC consortium is to build machine learning models and data flows. They help to predict inflow to a wastewater treatment plant in Copenhagen.
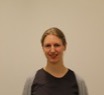
That’s why I liked the article ‘The ML Test Score: A Rubric for ML Production Readiness and Technical Debt Reduction‘ (Breck et al. 2017). The authors propose a systematic way of assessing how robust a machine learning system is. It is often the case that machine learning projects in organisations are done on local laptops but rarely deployed.
To make the most of the growing amounts of data and the advantages of data-driven approaches, it is essential that machine learning projects get put to work in the real world. This is only possible if data-based systems operate reliably. The article suggests a systematic way to discover weaknesses.